Summary:
UCLA researchers in the Department of Radiological Sciences have developed a novel neural network that can segment and quantify visceral and subcutaneous adipose tissues on magnetic resonance images automatically, accurately, and rapidly, to enhance clinical methods for characterizing and monitoring risk for cardiometabolic diseases in patients with obesity.
Background:
According to the Centers for Disease Control and Prevention, obesity affects roughly 42% of adults and 20% of children ages 2 to 19, and costs the U.S. healthcare system a staggering $173 billion of annual medical spending. Much of this cost is due to treatment of obesity-associated comorbidities, such as diabetes and hypertension. Mounting evidence highlights the role of adipose tissue, which is elevated in patients with obesity, in the development of these cardiometabolic diseases. Consequently, visceral adipose tissue (VAT) and subcutaneous adipose tissue (SAT) have been proposed as potential imaging biomarkers for detecting future risks for cardiometabolic diseases. Magnetic resonance imaging (MRI) can accurately quantify adipose tissue, but the current method for analysis, which requires intensive manual annotations, is laborious and requires expert training and knowledge. While deep learning-based methods have been investigated to automate VAT and SAT segmentation and quantification, the performance for VAT, which has been more strongly correlated with metabolic risk factors, remains inadequate due to the spatial variation and disconnected nature of VAT. Thus, a novel and facile method for segmentation of adipose tissue using MR images could help characterize risk factors and guide/monitor therapeutic interventions for cardiometabolic diseases in patients with obesity.
Innovation:
UCLA researchers led by Dr. Holden Wu in the Department of Radiological Sciences have developed a 3D neural network to automatically, accurately, and rapidly segment VAT and SAT from MRI. Their method uses combined multi-contrast MR images as inputs and a more sophisticated loss function for training. Using MRI scans from 629 participants, all of whom had notably high body mass index (BMI), indicating being overweight or obese, the researchers demonstrated that the method could successfully segment SAT and VAT. This method can be extended to segment SAT into two compartments, superficial and deep SAT, as well as brown adipose tissue (BAT) and epicardial adipose tissue (EAT). The method was also successfully tested to segment SAT and VAT on free-breathing abdominal MRI scans obtained from children. Taken together, a novel neural network for adipose tissue segmentation could advance the utility of MRI for characterizing risk for cardiometabolic diseases.
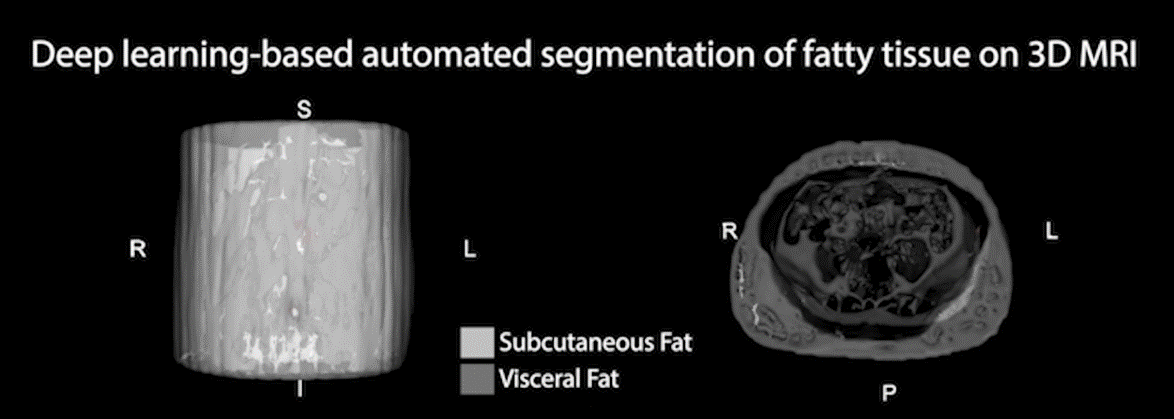
3D rendering of the subcutaneous and visceral fat segmentation results using the proposed 3D neural network with MRI inputs.
Potential Applications:
• A rapid automated tool to analyze the body composition of individuals with overweight or obesity
• Characterize risk for cardiometabolic diseases, including diabetes, elevated glucose levels, and hypertension
Advantages:
• Framework has two settings that allow for use even with limited training data and computational resources
• High performance due to emphasis of specific features in spatial and channel dimensions
• Improved segmentation performance of VAT
Development to Date:
The researchers have demonstrated successful use of their invention to segment subcutaneous and visceral adipose tissue on MRI scans taken from 629 adults and 34 children.
Related Papers (from the inventors only):
Kafali, S.G., Shih, SF., Li, X. et al. Automated abdominal adipose tissue segmentation and volume quantification on longitudinal MRI using 3D convolutional neural networks with multi-contrast inputs. Magn Reson Mater Phy 37, 491–506 (2024). https://doi.org/10.1007/s10334-023-01146-3
SG Kafali, S Shih, X Li, T Armstrong, KV Ly, S Ghahremani, KL Calkins, HH Wu. Fully Convolutional Networks for Adipose Tissue Segmentation Using Free-Breathing Abdominal MRI in Healthy and Overweight Children, Annual Meeting of International Society of Magnetic Resonance in Medicine (ISMRM), Virtual conference, Program Number 0071, August 2020.
SG Kafali, S Shih, X Li, T Armstrong, K Kuwahara, S Govardhan, KV Ly, S Ghahremani, KL Calkins, HH Wu. A Densely Connected Neural Network with Frequency Balancing Loss for Adipose Tissue Segmentation in Children using Free-Breathing Abdominal MRI, Annual Meeting of International Society of Magnetic Resonance in Medicine (ISMRM), Virtual conference, Program Number 2263, May 2021.
SG Kafali, S Shih, X Li, S Chowdhury, S Loong, S Barnes, Z Li, HH Wu. 3D Neural Networks for Visceral and Subcutaneous Adipose Tissue Segmentation using Volumetric Multi-Contrast MRI, 43rd Annual International Conference of the IEEE Engineering in Medicine and Biology Society (IEEE EMBS), November 2021.
SG Kafali, S Shih, X Li, S Chowdhury, S Loong, S Barnes, Z Li, HH Wu. Automated Adipose Tissue Segmentation using 3D Attention-Based Competitive Dense Networks and Volumetric Multi-Contrast MRI, Annual Meeting of International Society of Magnetic Resonance in Medicine (ISMRM), London, Program Number 0553, May 2022.