Summary:
UCLA researchers in the Department of Electrical and Computer Engineering have developed an all-optical diffractive network that can find applications in machine learning-related vision tasks and all-optical computing systems.
Background:
Optical computing is a gateway to the future, as it can revolutionize computation by making classically stubborn problems solvable. Compared to classical computing schemes, an optical computing system requires little to no power to operate. At this moment, countless computing devices that store and process unprecedented amounts of data are consuming non-renewable power heavily. In 2019, the electric power consumed by the global data centers reached around 190 billion kilowatt-hours, and the data centers in the U.S. consumed approximately 40% of it. Another key factor fueling the growing interest in optical computing is the massive progress achieved in artificial intelligence. Together with transformative hardware advances—including innovations in autonomous driving, remote sensing, robotic systems, smart homes, surveillance, and the Internet of Things—the development of practical optical computing systems represents a paradigm shift in machine learning-related tasks, especially for visual computing applications. Despite many research works conducted, currently, no optical computing system is matured enough to perform computations that traditional computers could. In order to quickly complete future artificial intelligence tasks that require low-power execution, there is a clear need for a novel computing framework.
Innovation:
Professor Ozcan and his research team have invented a single diffractive network that is operated optically to enable multiple arbitrarily-selected linear transformation operations with complex values. In this all-optical system, the computational task is completed as the light passes through thin and passive optical elements, so the execution is performed at the speed of light, and the process does not consume power except for the illumination light. It utilizes standard isotropic diffractive materials, which make the fabrication cost-effective and scalable. Compared to traditional methods to employ multiple diffractive subsystems, this innovation applies polarization multiplexing in a single diffractive network, and integrates multiple tasks within the same system, rendering it better in terms of speed, versatility, and compactness. Additionally, the computing capacity of this diffractive network can be improved further, as the polarization multiplexing can be flexibly coupled with other multiplexing methods.
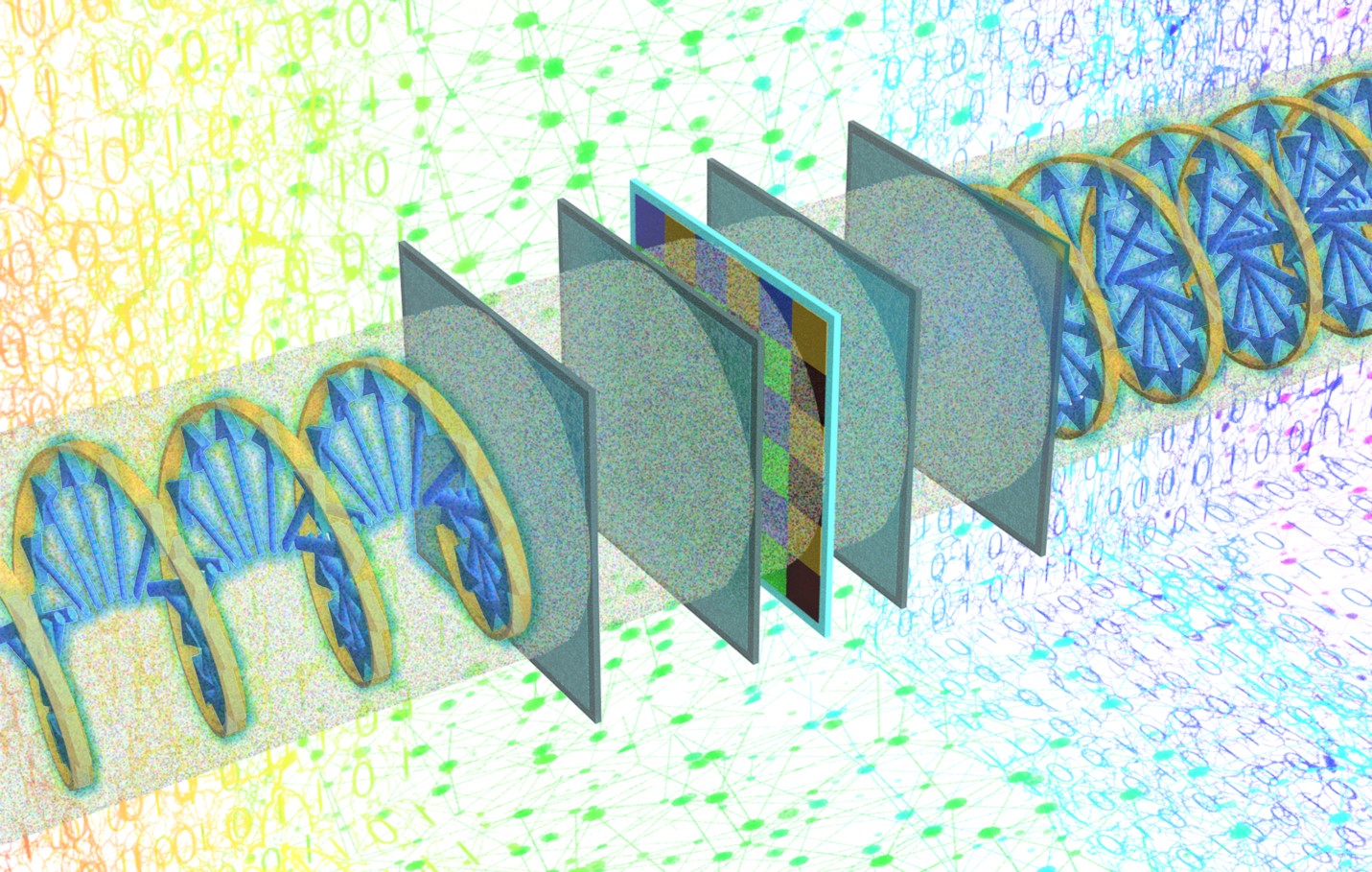
Potential Applications:
• All-optical high-throughput processors
• Machine vision computing systems
• Polarization-aware optical information processing systems
• Artificial intelligence tasks
• High-performance general-purpose processing systems
• Optical information processing (e.g., object classification & image reconstruction)
• Quantitative phase imaging
Advantages:
• Processing at the speed of light
• Low-power execution
• Simple design and common materials selection
• Easy fabrication with great scalability
• Versatile, compact, and cost-effective system
Development to Date:
First successful demonstration (first actual reduction to practice): July/2021.
Related Papers:
Li, J., Hung, Y.C., Kulce, O., Mengu, D. and Ozcan, A., 2022. Polarization multiplexed diffractive computing: all-optical implementation of a group of linear transformations through a polarization-encoded diffractive network. Light: Science & Applications, 11(1), pp.1-20.
Lin, X., Rivenson, Y., Yardimci, N.T., Veli, M., Luo, Y., Jarrahi, M. and Ozcan, A., 2018. All-optical machine learning using diffractive deep neural networks. Science, 361(6406), pp.1004-1008.
Reference: UCLA Case No. 2022-230
Lead Inventor: Prof. Aydogan Ozcan.