Summary:
UCLA researchers in the Department of Electrical and Computer Engineering have developed a novel camera design that is capable of target-specific imaging with instantaneous optical erasure of irrelevant objects.
Background:
There are over 1 billion surveillance cameras around the world today, and the installation of such video surveillance technology will continuously grow due to increasing adoption of automation systems in public and private settings. In this digital era, privacy protection has become a rising problem. Moreover, the demand for digital computing power and data storage space is constantly growing to support the processing of massive amounts of visual data gathered every day. The basic and most commonly adopted image processing methods, such as image blurring, encryption, and deep learning-based algorithms, address privacy concern with software-based techniques. The intrinsic flaw of these techniques is that the sensitive information is only preserved after the recording and transmission of the raw data. Data breaches can still easily occur during image digitization and transmission, especially when captured from remote devices. Another set of solutions are implemented at the hardware level, in which the data processing happens before data transmission. However, they do not completely address the privacy concern, and exposure of confidential information can still happen during the digitization process. There are methods that can enforce privacy before the image digitization, but they are not widely adopted because of poor quality of the generated images, and such methods limit the further application of captured images for downstream tasks, i.e., human pose estimation. There is a growing need for a novel camera design that can generate images with desired quality while ensuring privacy and promoting sustainability.
Innovation:
Professor Ozcan and his research team have invented a novel camera design that can intervene into the light propagation and image formation stage to passively enforce privacy before raw data is recorded. This innovation provides a desired solution to both privacy and environmental concerns. The imaging system is comprised of diffractive layers that are trained using state-of-the-art deep learning techniques and fabricated with a 3D printer. This innovation is able to perform optical mode filtering to accurately form images of the target objects, while simultaneously erasing objects of the irrelevant class at the output field-of-view. Applying the same idea, the design of class-specific permutation cameras is realized successfully to provide all-optical image encryption for the desired objects—providing an extra layer of data security. Additionally, this innovation can easily be scaled to different parts of the electromagnetic spectrum, ranging from the visible to infrared wavelengths, to open up new possibilities for task-specific imaging/video that is privacy-preserving and data-efficient.
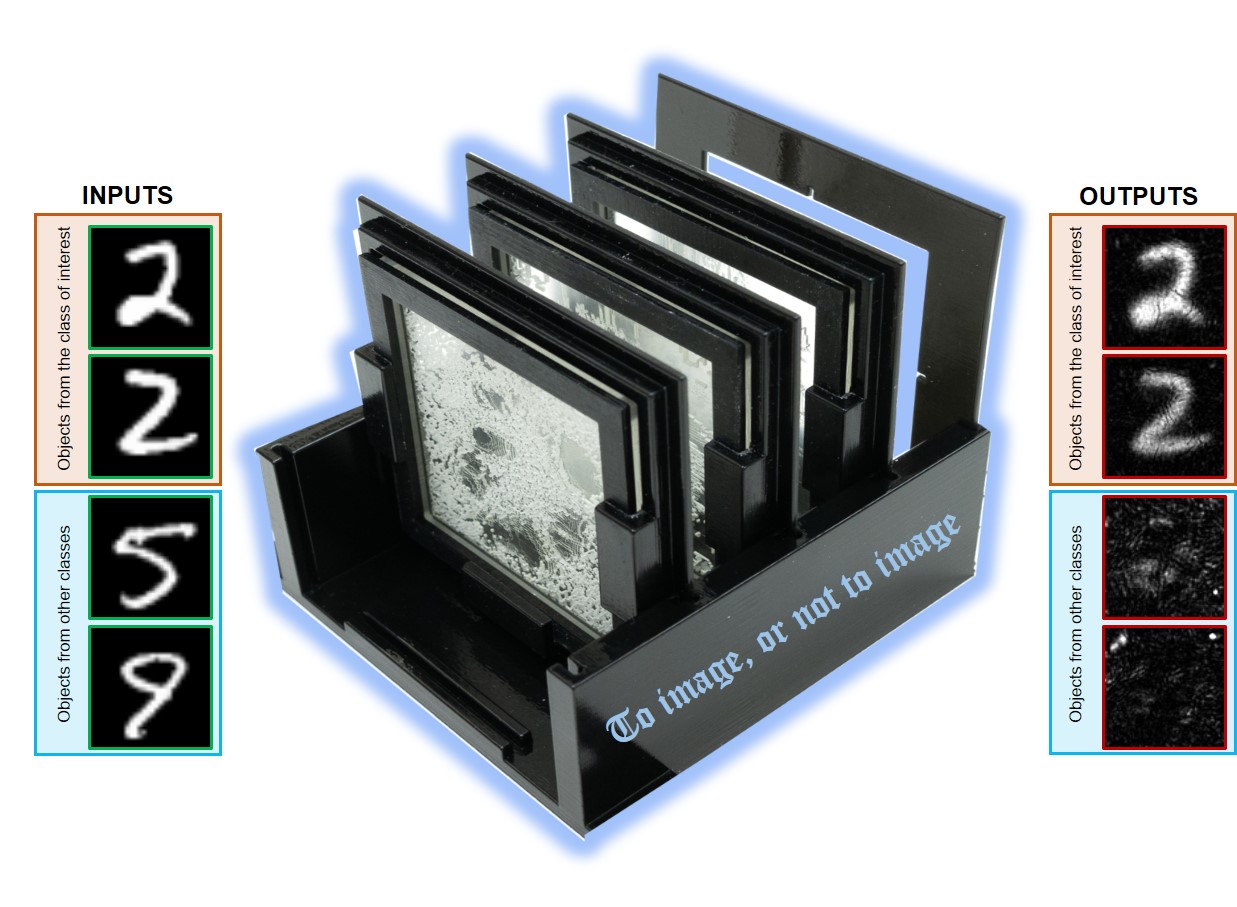
Potential Applications:
• Surveillance camera
• Live streaming camera
• Deployment on self-driving cars
• Image encryption
Advantages:
• Accurately captures target class with high fidelity
• Optically and instantaneously erases irrelevant objects
• Privacy protection
• Saves transmission load and digital storage space
• Transformative nature over the electromagnetic spectrum
• Easy fabrication using 3D-printing
• Environmentally-sustainable methodology
Development to Date:
First successful demonstration (first actual reduction to practice) has been completed: June, 2019.
Related Papers:
https://elight.springeropen.com/articles/10.1186/s43593-022-00021-3
https://doi.org/10.1186/s43593-022-00021-3
Reference:
UCLA Case No. 2022-287
Lead Inventors:
Dr. Aydogan Ozcan; Dr. Yi Luo; Dr. Bijie Bai