Summary:
UCLA researchers in the Department of Electrical and Computer Engineering have developed an air analysis instrument and accompanying virtual aerosol detection method that combines imaging and deep learning to sense and classify airborne particles without external labeling or post processing steps.
Background:
Air quality management, particularly the detection and classification of bio-aerosols, is integral to safeguarding human health, with the global surge in respiratory illnesses and increasing pollen allergies underscoring its importance. Traditional methods for analyzing airborne particulates involve sampling followed by time-consuming and often expensive expert scrutiny, hindering real-time insights and broader accessibility. While newer techniques such as chemical labeling and specialized filtration have improved availability, they compromise on collection sensitivity, efficiency, and scalability, further restricting throughput. Furthermore, these methods necessitate elaborate sample preparations and regular maintenance, adding layers of complexity. The crux of the challenge lies in devising a solution that combines high precision, prompt feedback, and user-friendliness in identifying a spectrum of airborne particles, from pollens to mold spores. Any innovative approach that addresses these prevalent shortcomings not only bridges the existing technological gap but also promises a transformative impact on air quality monitoring and related health interventions.
Innovation:
UCLA researchers have developed a virtual impactor-based bio-aerosol detector. Virtual impactors are devices used to separate airborne particles into distinct airstreams based on their size. The use of a virtual impactor is coupled with lens-less computational imaging, sidestepping the need for filtration and maintenance. By leveraging a deep neural network, it achieves a >94% accuracy in classifying diverse pollen types. The system is compact and cost-effective to manufacture. Capture, imaging, and analysis is all done within a single, portable closed system, enabling real-time, long term air quality monitoring.
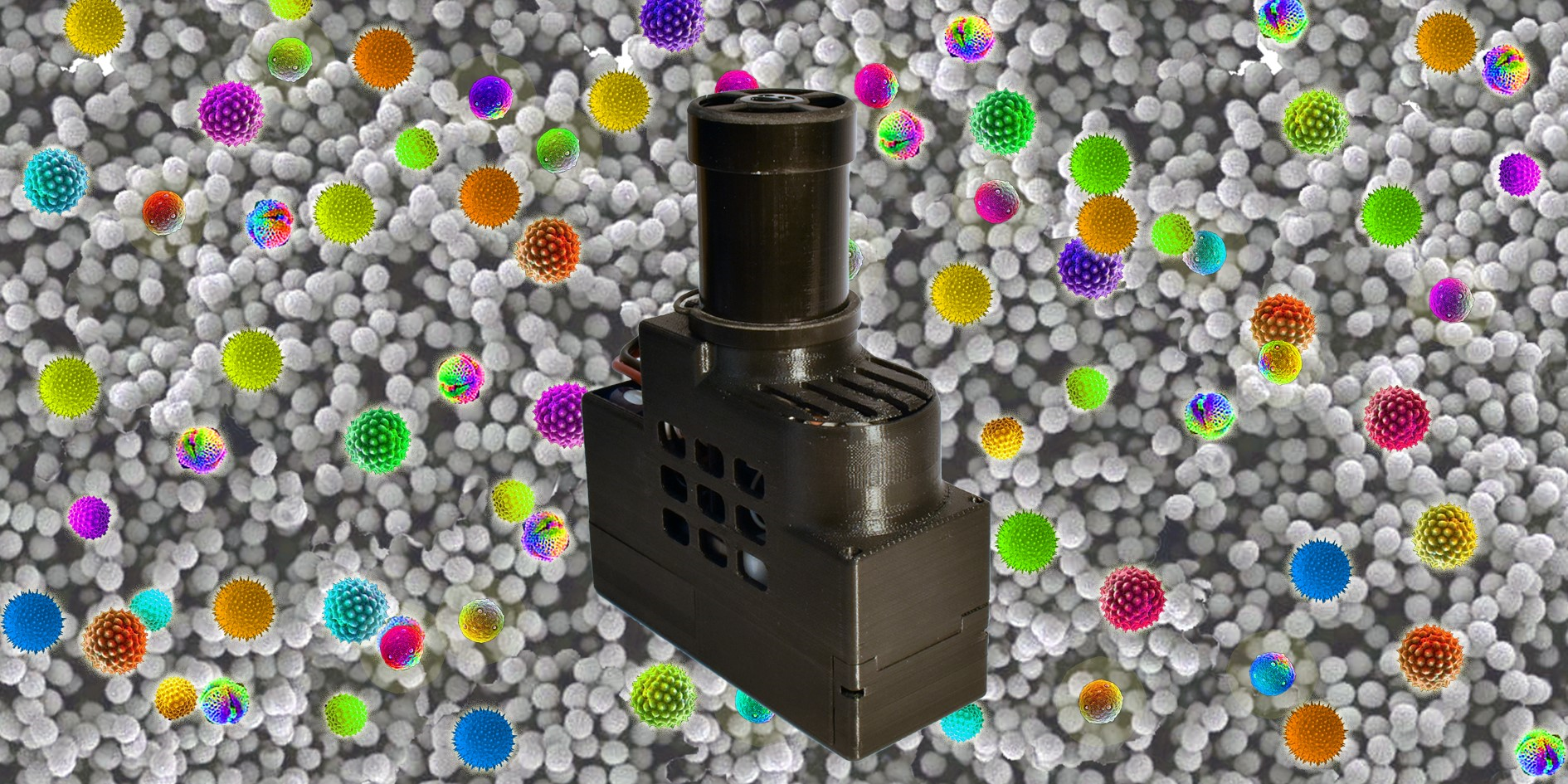
Credit: Ozcan Lab
Potential Applications:
• Indoor air quality monitoring
• Environmental monitoring
• Agricultural & plant biology research
• Industrial applications: food processing, fermentation
• Bioterrorism detection
Advantages:
• Cost effective
• Hand-held, portable
• Wireless control
• Label-free detection
• Extended, real-time monitoring
• >94% accuracy in identifying different pollen types.
State of Development:
The inventors have developed an instrument and accompanying software for the identification of airborne bio-pathogens. Accurate identification of six common pollen spores has been demonstrated and an article has been published in a research journal.
Related Papers:
1. Wu, Yichen, Ayfer Calis, Yi Luo, Cheng Chen, Maxwell Lutton, Yair Rivenson, Xing Lin et al. "Label-free bioaerosol sensing using mobile microscopy and deep learning." ACS Photonics 5, no. 11 (2018): 4617-4627.
2. Ozcan, Aydogan, and Yichen Wu. "Label-free bio-aerosol sensing using mobile microscopy and deep learning." U.S. Patent 11,262,286, issued March 1, 2022.
Reference:
UCLA Case No. 2019-722
Lead Inventor:
Aydogan Ozcan, UCLA Professor of Electrical and Computer Engineering