Summary:
UCLA researchers in the Department of Electrical and Computer Engineering have developed a virtual aerosol detection method that combines imaging and deep learning to sense and classify bio-aerosols without any external labeling or post processing steps.
Background:
Bio-aerosol detection and classification is pivotal to understanding and monitoring air quality, which has direct implications for human health, especially for those who suffer from extremely common pollen allergies. Conventional airborne particulate sensing consists of sampling and a secondary expert analysis. The secondary analysis limits accessibility and real time sensing and reporting capabilities of the technology. Virtual impactors have been utilized to separate ambient particles based on their size, allowing researchers to detect the presence of chemicals such as aerosols. Recent advancements in the field include chemical labeling and filtration processes, which increase accessibility but limit collection sensitivity, efficiency, scalability, and throughput. When these methods are used for bio-aerosol detection, there is a need for additional steps such as culturing and PCR analysis, further limiting testing bandwidth. A significant challenge lies in the need for highly accurate, accessible methods to identify diverse airborne particles, like pollen, without the requirement of elaborate preparation or frequent maintenance.
Innovation:
UCLA researchers have developed a virtual impactor-based bio-aerosol detector. The use of a virtual impactor is coupled with computer imaging and a neural network for classification, removing the need for any maintenance-necessitating filtration, secondary labeling, or external analysis. Multiple holographic images are captured of each particle, capturing volumetric information with a vast field-of-view; enabling higher classification accuracy and detailed visualization from multiple angles. By leveraging a deep neural network, it achieves a >92% accuracy in classifying diverse pollen types. The system is compact and cost-effective to manufacture. Capture, imaging, and analysis is all done within a single, closed system, enabling real-time, long term air quality monitoring. In addition, this technology can be utilized to monitor bacterial and viral particulates for a diverse array of applications.
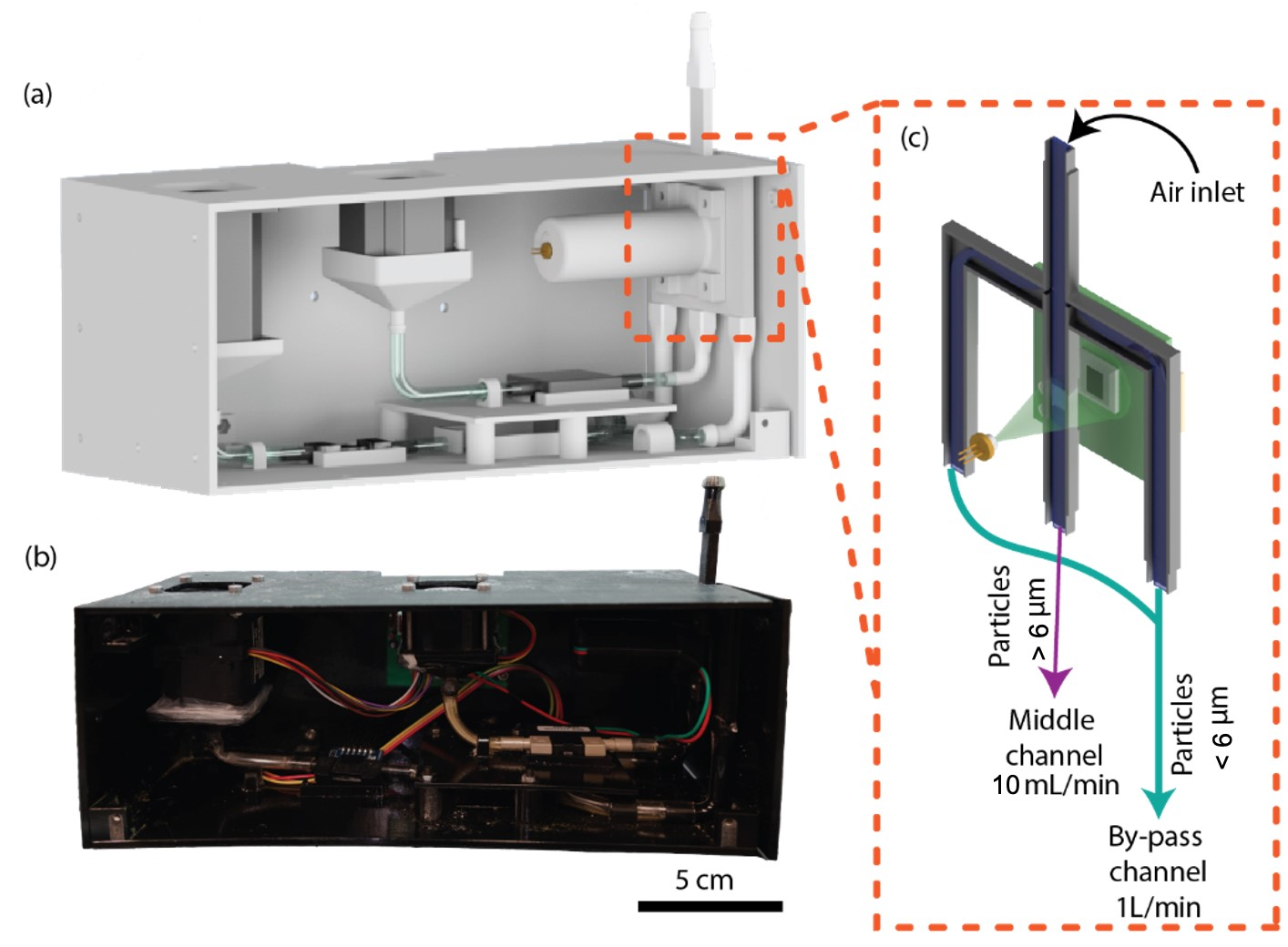
Potential Applications:
• Indoor air quality monitoring
• Environmental monitoring
• Agricultural & plant biology research
• HVAC system integration
• Viral or bacterial monitoring
• Bioterrorism defense
Advantages:
• Low cost
• Portable
• Label-free detection
• Extended, real-time monitoring
• ~93% accuracy in identifying different pollen types
State of Development:
The inventors have developed an instrument and accompanying software for the identification of airborne bio-pathogens. Accurate identification of six common pollen spores has been demonstrated and an article has been published in a research journal.
Related Publications:
- Luo, Y., Zhang, Y., Liu, T., Yu, A., Wu, Y. and Ozcan, A., 2022. Virtual impactor-based label-free pollen detection using holography and deep learning. ACS sensors, 7(12), pp.3885-3894. https://pubs.acs.org/doi/10.1021/acssensors.2c01890
Reference:
UCLA Case No. 2023-037
Lead Inventor:
Aydogan Ozcan, UCLA Professor of Electrical and Computer Engineering